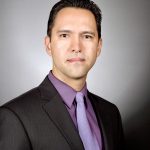
Title: Associate Professor, University of Texas at Rio Grande Valley
Dr. Kim aims to highlight their extensive knowledge and contributions in various areas of research. One of his key focuses lies in humanoid locomotion simulation using Reinforcement Learning (RL). Through the utilization of RL techniques, his research endeavors revolve around training a humanoid or musculoskeletal model to accurately replicate human motion. By leveraging the power of RL, Dr. Kim seeks to enhance the capabilities and efficiency of humanoid robots, enabling them to perform complex locomotion tasks with human-like dexterity and adaptability.
Additionally, he has delved into the realm of material science, particularly in the prediction of properties for High Entropy Alloys (HEAs). Employing Deep Neural Networks (NN) and Generative Adversarial Networks (GANs), his research explores the potential of these advanced machine learning techniques in accurately forecasting and predicting the properties of HEAs. This work contributes to the advancement of materials engineering and opens avenues for the development of novel alloys with superior properties.
Furthermore, he has also worked on structure-based virtual screening for drug discovery utilizing RL methodologies. By combining RL algorithms with structural biology approaches, his research aims to expedite the identification of potential drug candidates by efficiently screening vast libraries of compounds. This interdisciplinary work has the potential to revolutionize the drug discovery process, leading to the development of new therapeutic interventions for various diseases.
Beyond these areas, his research interests extend to diverse fields, including the development of a honey bee monitoring system for ecological studies and conservation efforts. Moreover, he has explored the simulation of concrete conductivity, investigating its electrical properties to inform the design and optimization of concrete structures. Additionally, he has contributed to Rio Grande River Salinity Forecasting, employing data-driven models to predict and mitigate salinity levels in the river, thereby facilitating sustainable water resource management.
Overall, Dr. Kim's research expertise encompasses a wide range of topics, leveraging state-of-the-art techniques such as RL, NNs, GANs, and data-driven modeling to address complex challenges and contribute to the advancement of various fields.